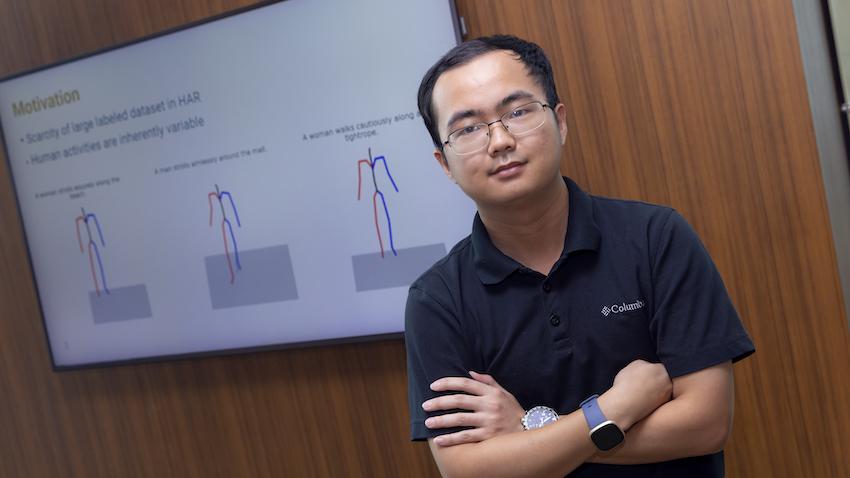
Student Shows ChatGPT Can Save Time, Resources for Sensory Data Researchers
A new data-gathering method could save sensory technology and human-activity recognition (HAR) researchers and data collectors a significant amount of time and money spent on resources.
In a recent paper, Georgia Tech third-year computer science major Zikang Leng introduced a large-language model approach with ChatGPT that could revolutionize how researchers collect sensory data.
Leng will present his paper this week at the 2023 Association of Computing Machinery’s joint conference on Pervasive and Ubiquitous Computing (UbiComp) in Cancun, Mexico. Generating Virtual On-body Accelerometer Data from Virtual Textual Descriptions for Human Activity Recognition is a best-paper nominee at the conference.
Data scientists and researchers gather sensory data for human-activity recognition to build wearable technology such as smartwatches and fitness trackers. Traditionally, this requires collecting hours of sensing data on human test subjects and then meticulously annotating that data on a massive scale.
“In the human activity recognition community, there’s the challenge of not having enough labeled data,” said Leng, who works in the Computational Behavior Analysis Lab directed by associate professor Thomas Ploetz. “To train a machine learning model that can recognize human activity based on sensory data, you need a lot of data. The labeling process is costly. You need to recruit and record human subjects, and you need a person to annotate the data. Thirty minutes of data could take 30 hours to annotate.”
In a recent paper, Georgia Tech third-year computer science major Zikang Leng introduced a large-language model approach with ChatGPT that could revolutionize how researchers collect sensory data.
Leng will present his paper this week at the 2023 Association of Computing Machinery’s joint conference on Pervasive and Ubiquitous Computing (UbiComp) in Cancun, Mexico. Generating Virtual On-body Accelerometer Data from Virtual Textual Descriptions for Human Activity Recognition is a best-paper nominee at the conference.
Data scientists and researchers gather sensory data for human-activity recognition to build wearable technology such as smartwatches and fitness trackers. Traditionally, this requires collecting hours of sensing data on human test subjects and then meticulously annotating that data on a massive scale.
“In the human activity recognition community, there’s the challenge of not having enough labeled data,” said Leng, who works in the Computational Behavior Analysis Lab directed by associate professor Thomas Ploetz. “To train a machine learning model that can recognize human activity based on sensory data, you need a lot of data. The labeling process is costly. You need to recruit and record human subjects, and you need a person to annotate the data. Thirty minutes of data could take 30 hours to annotate.”