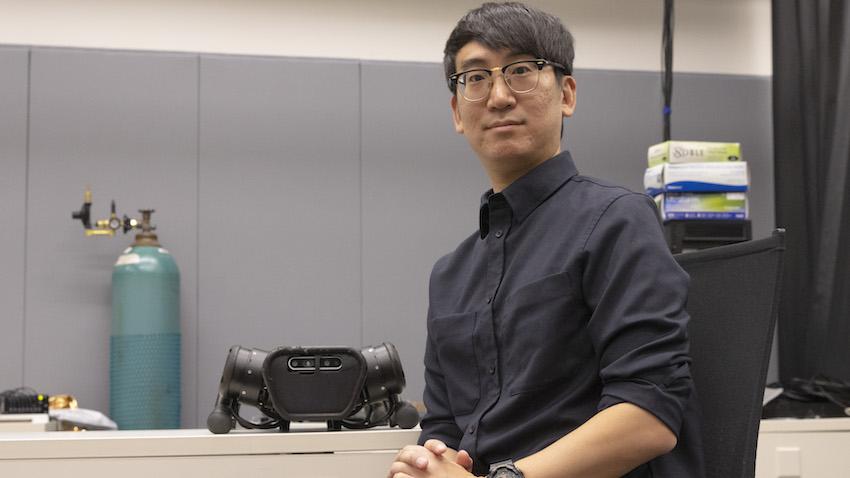
Two-World Training Approach Earns Roboticist NSF CAREER Award
Through his novel approach to combine simulation data with real-world ground truth data to train more capable robots, Assistant Professor Sehoon Ha has earned the National Science Foundation (NSF) CAREER Award.
The award is one of the most prestigious honors a junior faculty member can be given and typically comes with five years of funding for their proposed research.
Machine learning has opened the door for robots to enter the real world and learn about their environments and how to function within them with no pre-training data.
But having access to the ground-truth data for artificial intelligence isn’t a luxury that all robots have access to. For some robots, such as heavy-duty manufacturing machines or self-driving vehicles, it would be dangerous to introduce them to real-world environments and let them operate on trial and error.
The award is one of the most prestigious honors a junior faculty member can be given and typically comes with five years of funding for their proposed research.
Machine learning has opened the door for robots to enter the real world and learn about their environments and how to function within them with no pre-training data.
But having access to the ground-truth data for artificial intelligence isn’t a luxury that all robots have access to. For some robots, such as heavy-duty manufacturing machines or self-driving vehicles, it would be dangerous to introduce them to real-world environments and let them operate on trial and error.