Upcoming Events
A Decade of Machine Learning Accelerators: Lessons Learned and Carbon Footprint
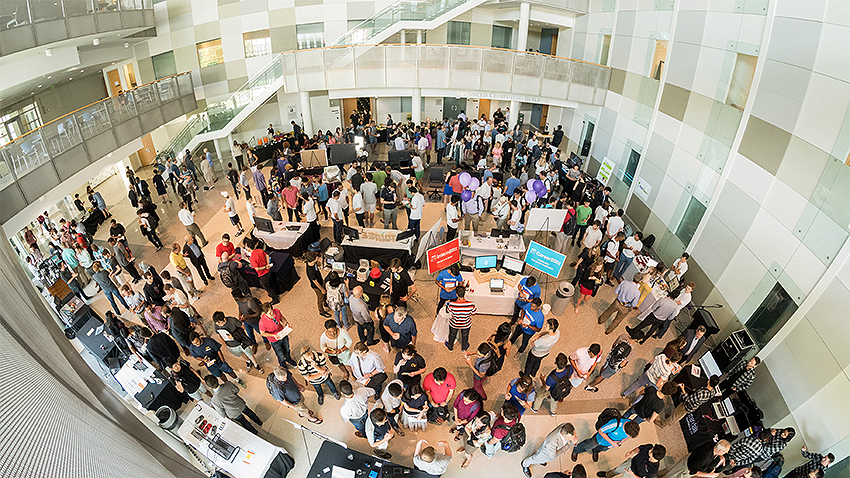
David Patterson, professor-emeritus at UC Berkeley and a Google distinguished professor, will give a lecture, A Decade of Machine Learning Accelerators: Lessons Learned and Carbon Footprints, on October 12. The talk will take place at 11 am with a question-and-answer session at 11:40, and it will be in rooms 1116-1118 of the Marcus Nanotechnology Building.
The success of deep neural networks (DNNs) from Machine Learning (ML) has inspired domain specific architectures (DSAs) for them. Google’s first generation DSA offered 50x improvement over conventional architectures for ML inference in 2015. Google next built the first production DSA supercomputer for the much harder problem of training. Subsequent generations greatly improved performance of both phases. We start with ten lessons learned from such efforts.
The rapid growth of DNNs rightfully raised concerns about their carbon footprint. The second part of the talk identifies the “4Ms” (Model, Machine, Mechanization, Map) that, if optimized, can reduce ML training energy by up to 100x and carbon emissions up to 1000x. By improving the 4Ms, ML held steady at <15% of Google’s total energy use despite it consuming ~75% of its floating point operations. With continuing focus on the 4Ms, we can realize the amazing potential of ML to positively impact many fields in a sustainable way.
David Patterson is a UC Berkeley Pardee professor emeritus, a Google distinguished engineer, and the RISC-V International Vice-Chair. His most influential Berkeley projects likely were RISC and RAID. His best-known book is Computer Architecture: A Quantitative Approach. He and his co-author John Hennessy shared the 2017 ACM A.M Turing Award and the 2022 NAE Charles Stark Draper Prize for Engineering. The Turing Award is often referred to as the “Nobel Prize of Computing” and the Draper Prize is considered a “Nobel Prize of Engineering.”
Event Details
Media Contact
Professor Tom Conte
conte@gatech.edu
EVENTS BY SCHOOL & CENTER
School of Computational Science and Engineering
School of Interactive Computing
School of Cybersecurity and Privacy
Algorithms and Randomness Center (ARC)
Center for 21st Century Universities (C21U)
Center for Deliberate Innovation (CDI)
Center for Experimental Research in Computer Systems (CERCS)
Center for Research into Novel Computing Hierarchies (CRNCH)
Constellations Center for Equity in Computing
Institute for People and Technology (IPAT)
Institute for Robotics and Intelligent Machines (IRIM)